Momotaz Begum

Research Projects
Human-Robot Collaboration in Special Education: A Robot that Learns Service Delivery from Teachers' Demonstrations
Location: UMass Lowell [2015 - present]
Sponsor: National Science Foundation
A significant amount of robotics research over the past decade showed that many children with autism spectrum disorders (ASD) have a strong interest in robots/robotic toys and concluded that robots are potential intervention tools for children with ASD. The clinical community and educators, who have the authority to approve robots in special education, however, are not convinced about the potential of robots. One major reason behind this gap is that the robotics research in this domain does not have a strong focus on the effectiveness of robots. This research aims to bridge the gap by designing a learning from demonstration (LfD) framework for robot-mediated special education service delivery for children with ASD and other disorders of a similar nature. The LfD framework will enable a robot to learn service delivery from a series of demonstrations by human educators in real special education scenarios. A multi-modal activity recognition framework will leverage the LfD framework by providing information about responses and activities of children with special needs as they engage with the robot. When the human educator has sufficient confidence in the robot’s ability, s/he will start collaborating with the robot in service delivery. In a collaborative service delivery scenario, the robot will autonomously deliver different steps of a specific educational service but will ask for the educator’s assistances in cases where it has low confidence in its perception and/or planned actions.
We are using Aldebaran's NAO robot for this research. We have already completed a 8 week-long user studies with five autistic childern at the Crotched Mountain School, NH. The data collected from the user study are currently being used to design a preliminary prototype of the proposed LfD framework.
Related Publications:
1. M. Begum, R. Serna, and H. A. Yanco, Are Robots Ready to Deliver Autism Interventions?A Comprehensive Review, International Journal of Social Robotics, 2016, DOI 10.1007/s12369-016-0346-y
2. M. Begum, R. Serna, D. Kontak, J. Allspaw, J. Kuczynski, J. Suarez, and H. Yanco, Measuring the efficacy of robots in ASD therapy: How informative are standard HRI metrics?, ACM/IEEE International Conference on Human-Robot Interaction, Portland, 2015
3. M. Begum, H. Yanco, R. Serna, D. Kontak, Robots in clinical settings for therapy of individuals with autism: Are we there yet?, IEEE IROS Workshop on Rehabilitation and Assistive Robotics (Organizers: B. Argall and S. Srinivasa ), 2014

Nao
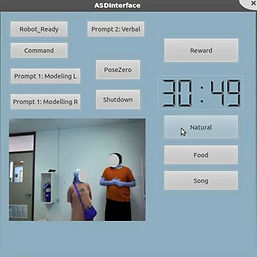
The robot control interface for collecting demonstration data

An example of a robot-mediated autism intervention session
2010 - present
2010 - present
Home-based Neuromoto Rehabiliation Using Werable Technologies
Location: University of New Hampshire, UMass Lowell [2015 - present]
Sponsor: IEEE-RAS SIGHT and National Science Foundation
The goal of this project is to design an intelligent framework for home-based neuorehabilitation for children with cerebral palsy. We are using two commercially available
wearable devices - a Myo armband and a pair of augmented reality eyeglasses - and advanced machine learning algorithms to design a framework which will enable children with cerebral palsy to perform rehabilitation exercises in the home/community settings. Our system uses a Markov decision process (MDP) model to learn individually-tailored exercises from a therapist’s demonstrations during the clinic visit of the patient. Electromyogrphy signals and IMU data from the patient are used for training the MDP model with
individually-tailored exercises. The trained model is used to control a 3D avatar that appears in the display screen of a pair of augmented reality eyeglasses (R-6 from Osterhout design group) to guide a patient to perform the exercise in the correct way. Such a system can ensure two factors key to achieve the expected motor recovery: accuracy in performing the exercise and frequency of performance.
Related Publications:
1. C. Munroe, Y. Meng, H. Yanco, and M. Begum, Augmented Reality Eyeglasses for Promoting Home-Based Rehabilitation forChildren with Cerebral Palsy, ACM/IEEE International Conference on Human-Robot Interaction, March 2016 (video demonstration)
2. Y. Meng, C. Munroe, Y. Wu, and M. Begum, A Learning from Demonstration Framework to Promote Home-basedNeuromotor Rehabilitation, IEEE International Symposium on Robot and Human Interaction Communication (Ro-Man), 2016
Personal Robots for Older Adults with Alzheimer's Dementia
Collaborator: Alex Mihailidis, Rosalie Wang, Rajibul Huq
Location: IATSL, Toronto Rehabilitation Institute [2012 -2013]
The long-term goal of this project is to design an autonomous assistive robot (AR) to help older adults with dementia (OAwD) in their activities of daily living (ADL). As a first step toward the user-centered design of such an assistive robot this project conducted a pilot study to investigate the feasbility of the idea of helping OAwDs through ARs and collected preliminary data regarding the users' needs and expectations. A prototype assistive robot 'ED' was built to conduct the pilot study where the robot 'ED' was tele-operated to provide need-based step-by-step guidance to 10 OAwDs (in presence of their caregivers) to complete two ADLs (tea-making and hand-washing) in a smart home. The study generated valuable information regarding the unique interaction patterns of the OAwDs, their specific requirements, and expectations from an assistive robot.
Related publications:
1. M. Begum, R. Huq, R. Wang, and A. Mihailidis, “Assisting Older Adults with Dementia: The Challenges in the Human-Robot Interaction Design for an Assistive Robot”, Gerontechnology, December 2013 (submitted)
2. M. Begum, R. Wang, R. Huq, and A. Mihailidis, “Performance of Daily Activities by Older Adults with Dementia: The Role of an Assistive Robot”, IEEE International Conference on Rehabilitation Robotics, Seattle, June 2013

Turn-Taking in HRI
Collaborator: Crystal Chao, Jinhan Lee, Andrea Thomaz
Location: Socially Intelligent Machine Lab, Georgia Tech [ 2010 -2011]
This research conducted a pilot study to collect information on turn-taking in a natural human-robot interaction scenario. During the study, Simon, an upper-torso humanoid robot from Meka, played 'Simon says' with 24 participants who has limited/no failiarity with robots. The turn-taking behavior of the participants were analyzed to design an automated model of turn-taking for HRI.
Related publications:
1. C. Chao, J. Lee, M. Begum, and A. Thomaz, “Simon plays Simon says: The timing of turn-taking in an imitation game”, IEEE International symposium on robot and human interactive communication (IEEE RO-MAN), pp. 235-240, 2011
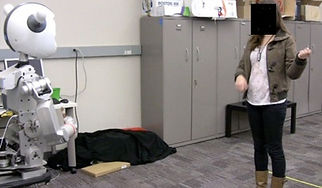
Self-directed Robot Learning
Collaborator: Fakhri Karray
Location: University of Waterloo, Canada [2007 -2010]
This project used the Bio-inspired Bayesian model of visual attention model to design a joint attention based human-robot interaction (HRI) framework for self-directed learning by robots. In the self-directed learning framework, a robot can manipulate the attention of its human partner (e.g. using hand gestures) and seek expert knowledge about an object of interest (e.g. by asking questions using natural speech). The framework enables a robot to learn only the information that the robot 'feels' is necessary to learn (in a given context). This reduces the burden on the human to organize rigorous training sessions to teach a robot with different objects/tasks/skills. A series of experiments shows that the robot performs significantly well in visual search of two test objects under significant affine transformation when it learned the objects' features (SIFT keypoints) through self-directed learning (visual search accurancy is 80%) as compared to the case when a human teacher choose the views of the objects for the robots to learn (visual search success is 47%).


Views of the objects chosen by a human teacher
that generated 47% success during a visual search

Related publications:
1. M. Begum and F. Karray, “Integrating visual exploration and visual search for robotic visual attention: The role of human-robot interaction," IEEE International Conference on Robotics and Automation (ICRA), pp. 3822- 3827, 2011 [nominated for the Best Cognitive Robotics Paper Award]
2. M. Begum, F. Karray, G. K. I. Mann, and R. G. Gosine, “A Probabilistic Approach for Attention-Based Multi-Modal Human-Robot Interaction”, IEEE International Symposium on Robot and Human Interactive Communication (IEEE RO-MAN), pp. 200-205, 2009
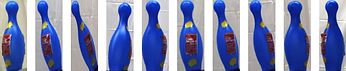
Views of the objects chosen by the robot itself that generated 80% success during a visual search
Collaborator: Fakhri Karray
Location: University of Waterloo, Canada [2007 - 2010]
A Bio-inspired Bayesian Model of Visual Attention for Robots
This research designed a bio-inspired visual attention model for robots. Inspired by the Biased Competitive hypothesis of primates' visual attention system, a Bayesian model of visual attention is designed that recursively estimates the head pose of a robot so that the robot can focuses on the stimuli in the environment that are the most relevant to the current behavioral state. The robot learns different stimuli (e.g. shapes, color, objects' name) attended throughout its life time and use those information to guide its visual attention behavior. The model enables the robot to execute a number of primates-like visual attention behaviors, e.g. visual search, visual exploration, inhibition of return, and novelty-habituation effect. A particle filter is used to implement the visual attention model on a robot with monocular and binocular vision.
Related publications:
1. M. Begum and F. Karray, “Robotic visual attention: A survey”, IEEE Transaction on Autonomous Mental Development, Vol. 3, No. 1, pp. 92-105, 2011
2. M. Begum, F. Karray, G. K. I. Mann, and R. G. Gosine, “A probabilistic model of overt visual attention for cognitive robots”, IEEE Transactions on System, Man, and Cybernetics B (SMC), Vol. 40, No. 5, pp. 1305-1318, 2010
3. M. Begum, F. Karray, G. K. I. Mann, and R. G. Gosine, “Re-mapping of Visual Saliency in Overt Attention: A Particle Filter Approach for Robotic Systems”, IEEE International Conference on Robotics and Biomimetics, pp. 425-430, 2009
4. M. Begum, G. K. Mann, R. Gosine, and F. Karray, “Object- and space-based visual attention: An Integrated Framework for Autonomous Robots”, IEEE/RSJ International Conference on Intelligent Robots and Systems, pp. 301-306, 2008
5. M. Begum, G. K. I. Mann, and R. G. Gosine, “A biologically inspired Bayesian model of visual attention for humanoid robots ”, Proceedings of IEEE-RAS International Conference on Humanoid Robots, pp. 587-592, 2006
Visual attention behaviors implemented with a mono-camera
Visual attention and pointing behavior implemented with a stereo-camera
Collaborator: George Mann, Raymond Gosine
Location: Memorial University, Canada [2003 -2005]
A Soft-Computing based SLAM Algorithm for Indoor Mobile Robots
This research developed a fuzzy-evolutionary range-based SLAM algorithm for indoor mobile robots. The proposed algorithm employs fuzzy logic to model the position uncertainty of a mobile robot and exploits the power of the island model genetic algorithm to conduct a stochastic search over the fuzzy-defined pose space for the most suitable robot position. Fuzzy rule-based inference system performs significantly well to model the subjective uncertainties in the robot's position arising from terrain anomalies, slippage, and other systematic errors. The island-model genetic algorithm enables the SLAM algorithm to maintain multiple hypotheses about the position of the robot and thereby helps to minimize loop-closing errors. The performance of the algorithm was comparable to contemporary range-based SLAM algorithms when tested on a Pioneer 3AT mobile robot equipped with SICK LMS laser range finder to map moderately large indoor environments (e.g. 15mx15m loop). The significant characteristics of the proposed SLAM algorithm is its simple mathematics and intuitive reasoning that are inherited from the soft-computing techniques. The technical articles that describe the gradual development of the algorithm were cited over 73 times (Google scholar citation count) in different SLAM-related articles.
[Map dimension: 14mx14m]
Related publications:
1. M. Begum, G. K. I. Mann, and R. G. Gosine, “Integrated fuzzy logic and genetic algorithmic approach for simultaneous localization and mapping of mobile robots ”, Journal of Applied Soft Computing, Vol. 8, No. 1, pp. 150–165, 2008
2. M. Begum, G. K. I. Mann, and R. G. Gosine, “An evolutionary SLAM algorithm for mobile robots”, Advanced Robotics, Vol. 21, No. 9, pp. 1031–1050, 2007
3. M. Begum, G. K. I. Mann, and R. G. Gosine, “An evolutionary algorithm for simultaneous localization and mapping (SLAM) of mobile robots ”, Proceedings of IEEE/RSJ International Conference on Intelligent Robots and Systems, pp. 4066-4071, 2006
4. M. Begum, G. K. I. Mann, and R. G. Gosine, “A fuzzy-evolutionary algorithm for simultaneous localization and mapping of mobile robots”, Proceedings of IEEE Congress on Evolutionary Computation , pp. 1975-1982, 2006
5. M. Begum, G. K. I. Mann, and R. G. Gosine, “Concurrent mapping and localization for mobile robot using soft computing techniques”, Proceedings of IEEE/RSJ International Conference on Intelligent Robots and Systems, pp. 2266-2271, 2005